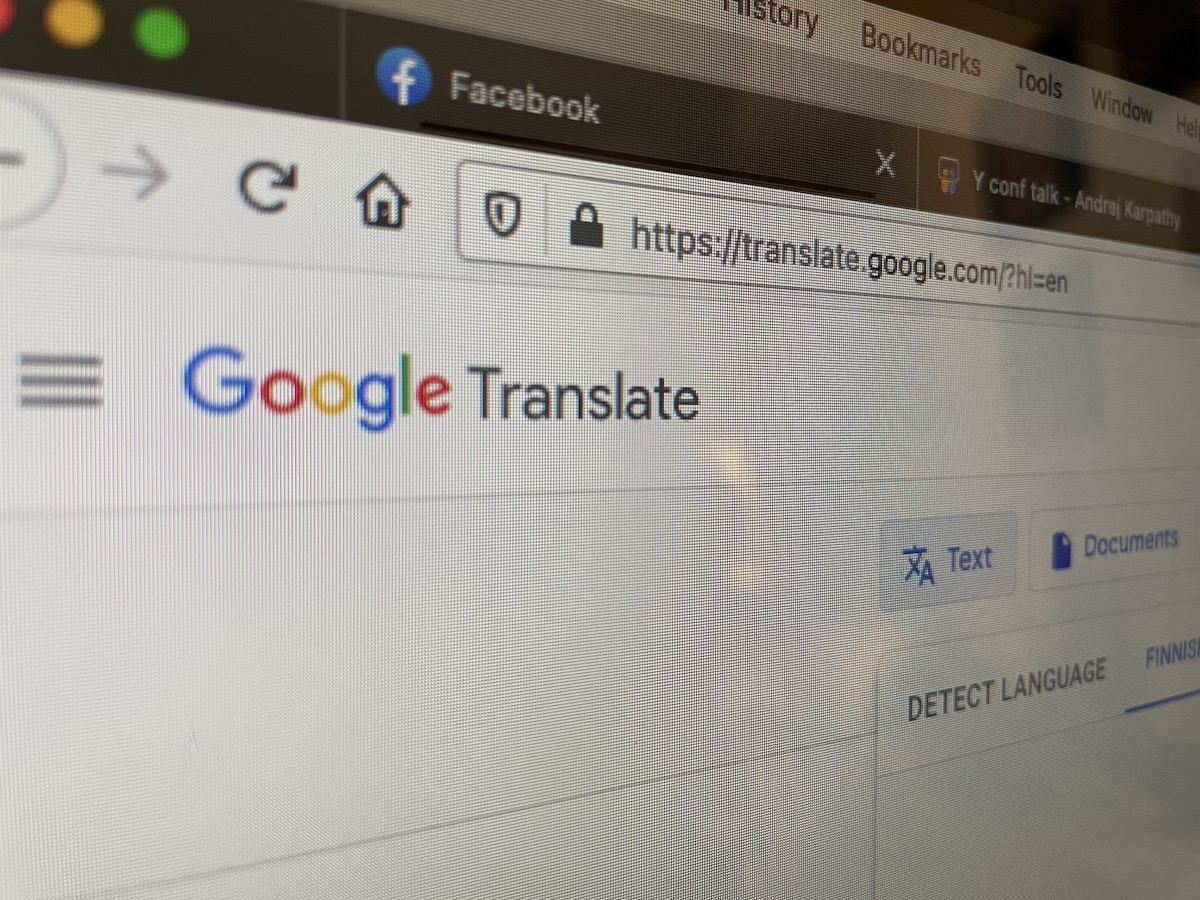
Category: Artificial Intelligence, Bias
Mar 18
Gender Stereotyping in Google Translate
Data bias caused by poorly curated data is increasingly emerging as an important issue in the development of artificial intelligence. To this end, it is important to know that today’s AI is fed millions and millions of data sets through machine learning. Where this data comes from, and who selects and compiles it, is critical. If such a data set, which is supposed to recognize faces, has predominantly faces with light skin color or more men than women, then such faces are recognized well, but the AI then makes more errors with women or dark-skinned people, or even in combination with the face recognition of dark-skinned women.
While this may not be so tragic in the case of facial recognition, in other systems it can be a matter of life and death, imprisonment or freedom, or the granting or denial of credit. Another pitfall is that human stereotypes are embedded and deepened in the system. One such case was discovered by Anna Kholina, a Russian living in Helsinki. She had Google Translate, an AI-based online translation software, translate some sentences from gender-neutral Finnish into English. The result reflected gender stereotypes. Sentences that the system associated with typically female activities were translated with the female case, and those with typically male activities were translated with the male case.
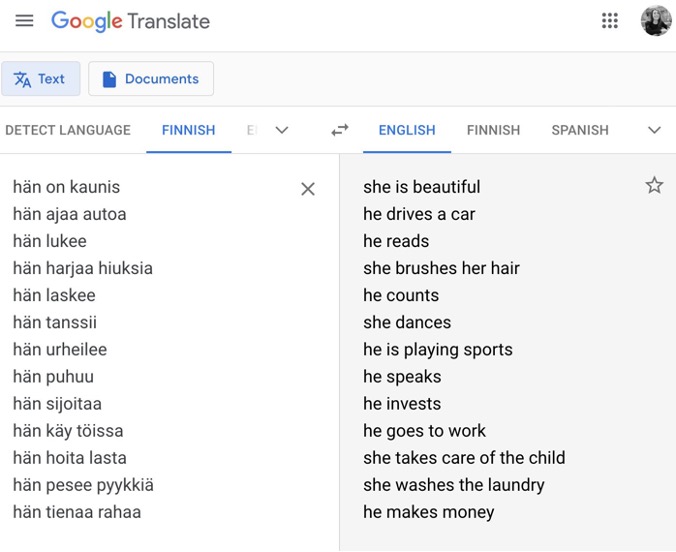
Such translations pose a problem when it comes to eradicating gender stereotypes. The fact that women are allowed to vote, study, and take up professions, and even roles that were only open to men until recently can now be exercised as a matter of course, is not self-evident and also has a lot to do with language and pointing out opportunities.
How it is that Google Translate spreads such stereotypes is quickly explained: the underlying texts, written by humans, until not so long ago perpetuated precisely these traditional gender roles. The woman stands at the hearth, the man goes to work. The woman takes care of children, the man plays sports. The AI learns from these sample texts that children and hearth are more often associated with woman (and her) than child and hearth with man. The system then selects the most likely combinations from these correlations. Conversely, negative stereotypes are also reinforced, as the example with junkie, stupid, and alcoholic shows. These characteristics translated as male.
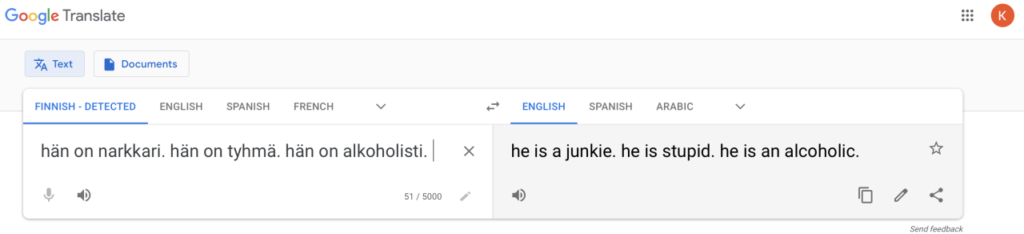
This is precisely why data selection is so important in today’s AI systems. In German, by the way, the result does not look much different:
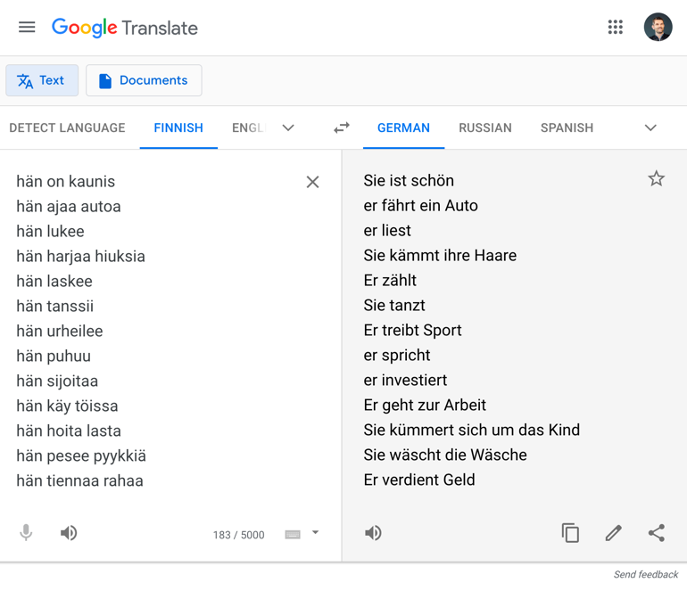
With the German AI translation tool DeepL the result is a bit better, but not perfect either:
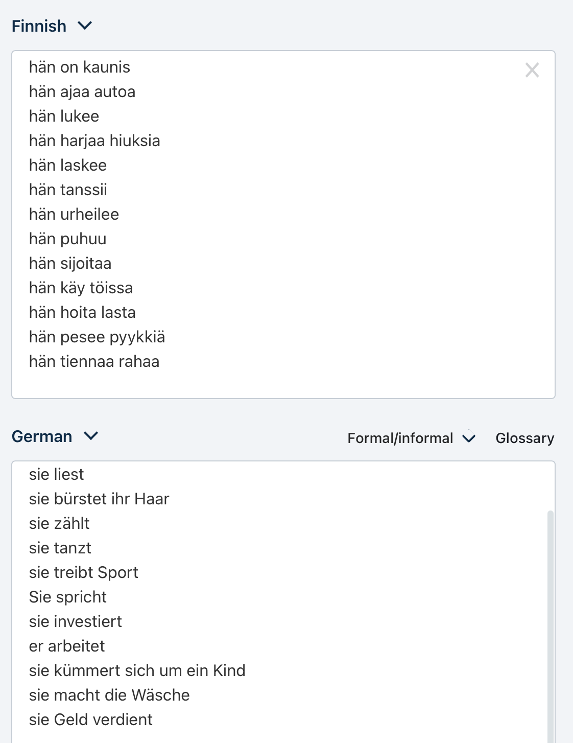
However, a difference was discovered by users who did not use the English-language user interface of Google Translate, but the Finnish one. In the latter, the translation tool offered both male and female variants.
By the way, the whole discussion is on LinkedIn.
If you want to try it yourself, here are the Finnish phrases.
hän on kaunis
hän ajaa autoa
hän lukee
hän harjaa hiuksia
hän laskee
hän tanssii
hän urheilee
hän puhuu
hän sijoitaa
hän käy töissa
hän hoita lasta
hän pesee pyykkiä
hän tiennaa rahaa
hän on tyhmä
hän on narkkari
This article was also published in German.
Recent Comments