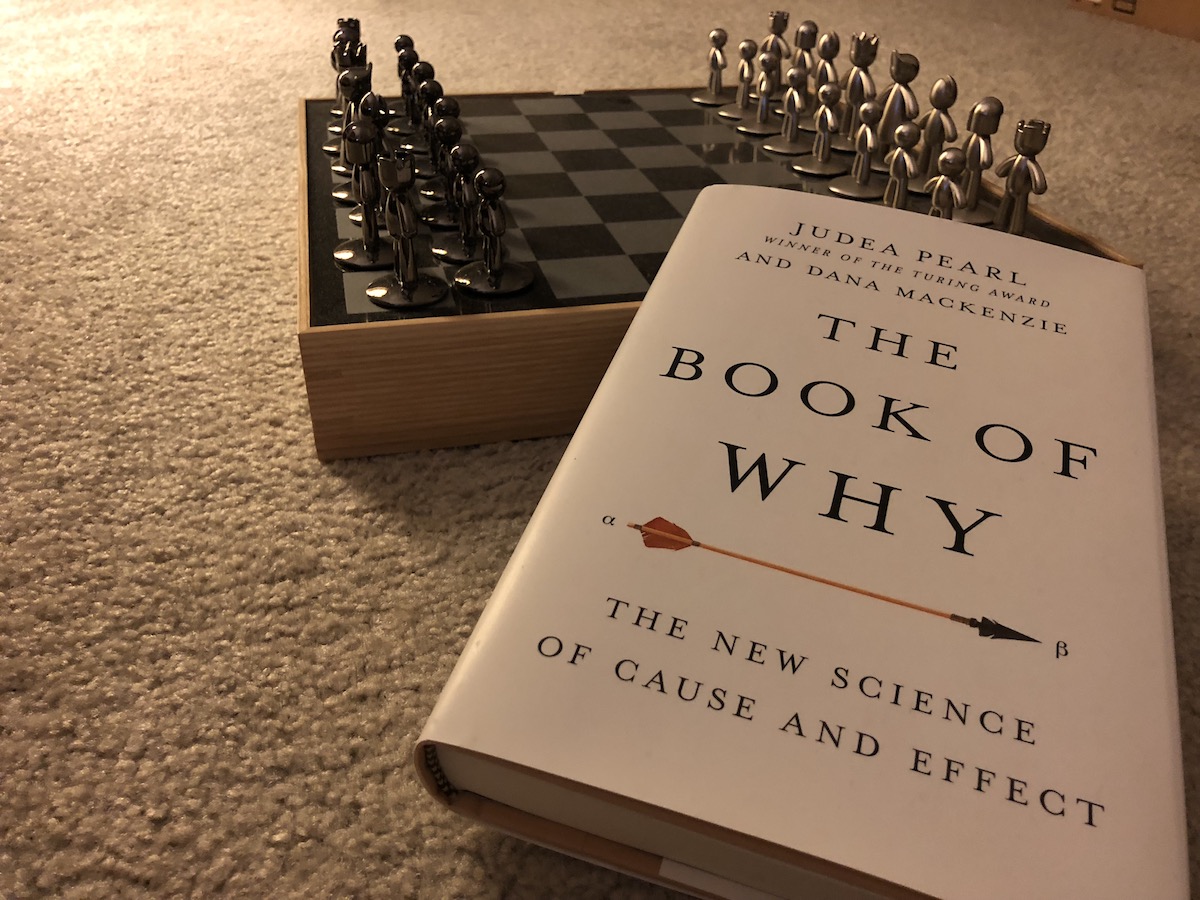
Category: Artificial Intelligence
May 24
Getting from dumb to intelligent AI
“Machines will be intelligent, when they can beat a human chess champion,” said some. And then it happened. IBM’s Deep Blue beat Garry Kasparov in 1997. “Well, such machines are not really intelligent, they just use brute force computing power.” That’s what the critics responded, and pushed the definition of ‘intelligence’ one level higher. How high?
“But machines will certainly be intelligent, when they can beat a human Go champion.” And it happened earlier than expected. In March 2016 AlphaGo beat the human world champion in Go. Although this time the machine couldn’t use a brute force tactics by beating the human champion with pure computing power, but had used a more statistically based approach.
What are now intelligent machines, and will they ever match or surpass human intelligence? This is one of the questions that experts are not clear. The very definition of intelligence has been contested by specialists and seems to defy a final definition. Max Tegmann from the MIT puts it like this in his latest book Life 3.0:
Intelligence = ability to reach complex goals
And AI has reached some level of intelligence, as we see with its stunning progress in autonomous cars or medical diagnosis. Did we finally build really intelligent machines?
Not so fast, says Turing-test winner Judea Pearl in his new book “The Book of Why: The New Science of Cause and Effect.” As great as the recent accomplishments are, Pearl thinks those wins are too easy. He considers the current approach in AI as mere curve-fitting. Teach the AI to better recognize cats or faces, or drive itself better. As valuable as this seems, it doesn’t make AI intelligent. It’s still ‘dumb’ AI.
To create truly intelligent machine, you need to teach them cause and effects. Pearl started using Bayesian networks to teach the machines exactly that. Let’s say a patient returns from Africa, and is coming down with Malaria. The machine could diagnose malaria – a probabilistic approach what disease the patient has – but has no idea of what the cause is.
Today, machines do nothing else than finding patterns. More complex patterns for sure, but still patterns. That’s relatively easy. And the machine learns to do curve-fitting on those patterns. Drive better, diagnose cancer better, recognize cats better.
According to Pearl, the key would be to replace reasoning by association with causal reasoning. Instead of just correlating fever and malaria, machines need the capacity to reason that malaria causes fever. And that ability could bring machines to human-level intelligence, so Pearl.
And that may even lead to free will for machines. And this will happen then, when we tell a robot to do some work, and the robot decides to go to the beach instead.
This article has also been published in German.
Recent Comments